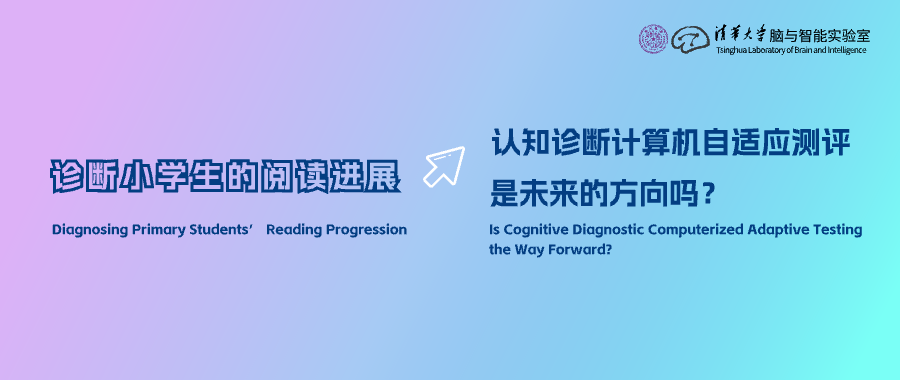
认知诊断计算机化自适应测评(CD-CAT)是教育测量领域的一项前沿技术,其目标是在提高测评准确性和效率的同时,对受测者的优缺点提供反馈。迄今为止,大多数 CD-CAT 研究都是在模拟条件下取得方法上的进展,但很少有人将 CD-CAT 应用于实际的教育评估。
据此,清华大学脑与智能实验室首席研究员刘嘉课题组展开了深入研究,利用 28485 名二至六年级学生的数据和项目级认知诊断模型(CDMs),系统比较了阅读 CD-CAT 系统的测量精度和效率,以满足认知诊断自适应和阅读能力发展监测的需求。
Abstract:
Cognitive diagnostic computerized adaptive testing (CD-CAT) is a cutting-edge technology in educational measurement that targets at providing feedback on examinees’ strengths and weaknesses while increasing test accuracy and efficiency. To date, most CD-CAT studies have made methodological progress under simulated conditions, but little has applied CD-CAT to real educational assessment. The present study developed a Chinese reading comprehension item bank tapping into six validated reading attributes, with 195 items calibrated using data of 28,485 second to sixth graders and the item-level cognitive diagnostic models (CDMs). The measurement precision and efficiency of the reading CD-CAT system were compared and optimized in terms of crucial CD-CAT settings, including the CDMs for calibration, item selection methods, and termination rules. The study identified seven dominant reading attribute mastery profiles that stably exist across grades. These major clusters of readers and their variety with grade indicated some sort of reading developmental mechanisms that advance and deepen step by step at the primary school level. Results also suggested that compared to traditional linear tests, CD-CAT significantly improved the classification accuracy without imposing much testing burden. These findings may elucidate the multifaceted nature and possible learning paths of reading and raise the question of whether CD-CAT is applicable to other educational domains where there is a need to provide formative and fine-grained feedback but where there is a limited amount of test time.
原文链接
the original paper link
https://doi.org/10.3102/10769986231160668
认知诊断计算机化自适应测评(CD-CAT)技术是目前最前沿的个性化智能测评技术之一。概括来说,CD-CAT充分结合了认知诊断评估和计算机化自适应测评技术的优势,可根据即时作答进行自适应估计和题目推荐,进而利用更少的题量、更短的时长,为学生提供更精确、更详细的优劣势诊断信息。如图1所示,认知诊断自适应测评的实现逻辑中包括了理论建构、题库开发与标定、校准模型、选题策略、曝光控制法、终止策略等重要环节,其在真实测评上的效果尚未可知。
本研究在前期《小学生阅读认知诊断测评》研究(Li et al., 2021)的基础上,进一步确定了小学2-6年级稳定存在的七种主要阅读属性掌握情况。这些主要的读者群及其随年级的变化表明,在小学阶段存在着某种逐步推进和深化的阅读发展机制。
此后,研究应用蒙特卡洛模拟了阅读CD-CAT系统的应用效果,比较了多种校准模型、选题策略、曝光控制法、终止策略等成分的表现,结果发现与随机选题和传统固定题本相比,CD-CAT有效地提高了模式水平的诊断信度和属性判准率,CD-CAT 在不增加测评负担的情况下显著提高了分类的准确性。
图3 多种标定模型与测评长度条件下CD-CAT测评精准度比较结果
本研究首次开发和验证了一套符合课程需求、有诊断功能、可监测发展的《小学生阅读认知诊断自适应测评》。测评系统的预期效果优于传统测评,具有较高的应用、落地价值,有助于实现学生阅读能力和技能的有效诊断。
这些发现可以阐明阅读的多面性和可能的学习路径,并提出了 CD-CAT 是否适用于需要提供形成性和精细反馈但测评时间有限的其他教育领域的问题。同时,研究还可围绕改进结果评价、强化过程评价、探索增值评价3个方面展开探索,为落实“减负增效”的教育改革提供有价值的参考依据。
本研究已在线发表于由美国教育研究协会主办的心理测量学权威期刊Journal of Educational and Behavioral Statistics,论文的第一作者为陕西师范大学教师发展学院(陕西教师发展研究院)的专任教师李燕博士,通讯作者为清华大学脑与智能实验室首席科学家、清华大学心理学系刘嘉教授。
相关研究
Li, Y., Huang, C., & Liu, J. (2023). Diagnosing Primary Students’ Reading Progression: Is Cognitive Diagnostic Computerized Adaptive Testing the Way Forward? Journal of Educational and Behavioral Statistics.
https://doi.org/10.3102/10769986231160668
Li, Y., Zhen, M., & Liu, J. (2021). Validating a Reading Assessment Within the Cognitive Diagnostic Assessment Framework: Q-Matrix Construction and Model Comparisons for Different Primary Grades. Frontiers in Psychology, 12, 5728.
https://doi.org/10.3389/fpsyg.2021.786612
部分参考文献
Carlson, S. E., Seipel, B., & McMaster, K. (2014). Development of a new reading comprehension assessment: Identifying comprehension differences among readers. Learning and Individual Differences, 32, 40–53.
https://doi.org/10.1016/j.lindif.2014.03.003
Chang, H. (2015). Psychometrics Behind Computerized Adaptive Testing. Psychometrika, 80(1), 1–20.Chang, H.-H., Wang, C., & Zhang, S. (2021). Statistical Applications in Educational Measurement. Annual Review of Statistics and Its Application, 8(1), 439–461.
https://doi.org/10.1146/annurev-statistics-042720-104044
Liu, H.-Y., You, X.-F., Wang, W.-Y., Ding, S.-L., & Chang, H.-H. (2013). The Development of Computerized Adaptive Testing with Cognitive Diagnosis for an English Achievement Test in China. Journal of Classification, 30(2), 152–172.
Ministry of education. (2011). the Chinese language curriculum criterion for compulsory education: 2011 edition. Beijing Normal University Press.
Ravand, H., & Baghaei, P. (2020). Diagnostic Classification Models: Recent Developments, Practical Issues, and Prospects. International Journal of Testing, 20(1), 24–56.
https://doi.org/10.1080/15305058.2019.1588278
Sorrel, M. A., de la Torre, J., Abad, F. J., & Olea, J. (2017). Two-Step Likelihood Ratio Test for Item-Level Model Comparison in Cognitive Diagnosis Models. Methodology, 13(Supplement 1), 39–47.
https://doi.org/10.1027/1614-2241/a000131
Sorrel, M. A., Nájera, P., & Abad, F. J. (2021). cdcatR: An R Package for Cognitive Diagnostic Computerized Adaptive Testing. Psych, 3(3), 386–403.
https://doi.org/10.3390/psych3030028
朱哲民, & 张华华. (2021). 认知诊断自适应测试的应用与展望. 中国考试, 01, 41–46.