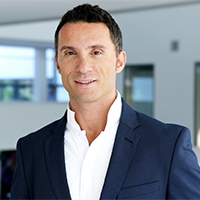
Carlo V. Cannistraci Prof. PhD. Eng.
Zhou Yahui Chair Professor
Chief Scientist, Tsinghua Laboratory of Brain and Intelligence (THBI)
Director, Center for Complex Network Intelligence (CCNI) at THBI
Department of Computer Science
Department of Biomedical Engineering
Tsinghua University, Beijing, China
If Physics studies the principles and mechanisms of the outside universe.
Brain science studies the principles and mechanisms of the inside universe.
My research is at the interface between these two disciplines.
I deal with "Physics and Engineering of Complexity and Intelligence":
studying principles of natural and artificial intelligence.
Carlo Vittorio Cannistraci Carlo Vittorio CaCarlo Vittorio Cannistraci
Biosketch
Dr. Cannistraci is a theoretical engineer and computational innovator. He is a Chair Professor in the Tsinghua Laboratory of Brain and Intelligence (THBI) and adjunct professor in the Department of Computer Science and in the School of Biomedical Engineering at Tsinghua University. He directs the Center for Complex Network Intelligence (CCNI) in THBI, which aims to create pioneering algorithms at the interface between information science, physics of complex systems, complex networks and machine intelligence, with a particular focus in brain/life-inspired computing for big data analysis. These computational methods are often applied to precision biomedicine, neuroscience, social and economic science.
Current research challenge of CCNI: Brain-network-inspired computing for next generation efficient and sustainable AI
The human brain is remarkably efficient, learning with just a few watts. In contrast, today’s large language models are voracious power consumers, demanding over 100 times the power expenditure of the human brain. Brain-inspired network science research can play a relevant role for designing low-consumption and efficient deep learning. We need to develop new concepts and theories for an ecological and sustainable approach to AI, and some of these new computing paradigms can be borrow from the brain and its complex systems biology.
In the Center for Complex Network Intelligence (CCNI), we are interested to investigate three key brain network features adopted for efficient computing: connectivity sparsity, connectivity morphology and neuro-glia coupling.
On sparsity, see our study “Epitopological learning and Cannistraci-Hebb network shape intelligence brain-inspired theory for ultra-sparse advantage in deep learning” is published in The Twelfth International Conference on Learning Representations (ICLR) 2024. On sparse morphology, see our study “Neuromorphic dendritic network computation with silent synapses for visual motion perception”2 is published in Nature Electronics.
Research strategy at CCNI
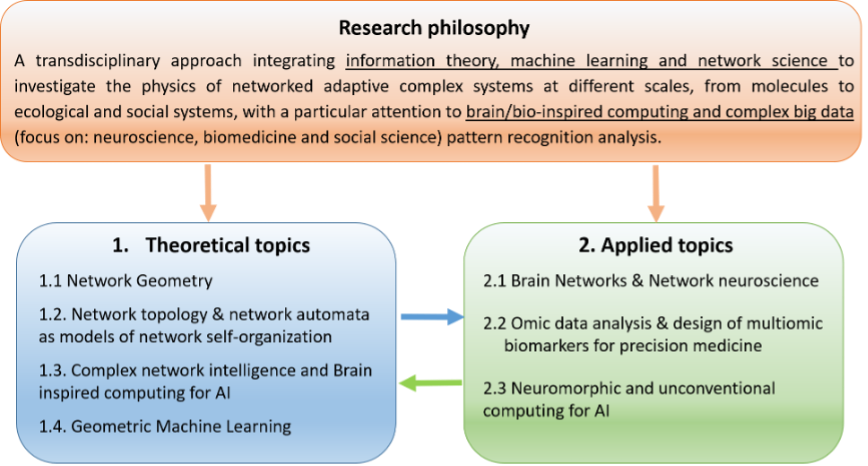
Organization chart of research at the Center for Complex Network Intelligence (CCNI). The chart clarifies what is the main strategy behind research at CCNI and how it influences the topics of our interest in theoretical and applied science. There is a clear interrelation between theoretical topics (which are crucial to offer new solutions in applied topics) and applied topics (which play an indispensable role to open new questions and to trigger innovation in theoretical research).
Research directions at CCNI
The CCNI adopts a transdisciplinary approach integrating information theory, machine intelligence and network science to investigate adaptive processes that characterize complex interacting systems at different scales, from molecules to ecological and socio-economic systems. This knowledge is leveraged to create novel and more efficient artificial intelligence algorithms; and to perform advanced analysis of patterns hidden in data, signals and images. Our theoretical effort is to translate advanced mathematical paradigms typically adopted in theoretical physics (like topology and manifold theory) to characterize many-body interactions in quantitative life science. We apply the theoretical frameworks we invent in the mission to develop computational tools for systems and network biology, personalized biomedicine and combinatorial drug therapy, social and economic data science.
Plasticity phenomena – like remodelling, growth and evolution – modify the topology of complex living systems, their internal state and their multidimensional representation in form of networks or high-dimensional datasets. Our theoretical mission is to elucidate the general rules and mechanisms that underlie this type of structural plasticity, which is at the basis of learning and memory processes in living organisms. In particular, we develop methods for topological analysis of self-adaptive and self-organizing learning systems such as protein interaction and bacteria-metabolite networks at the molecular level, and brain networks at the cellular level.
In neuroscience, we are interested in how the brain networks wire at synaptic and functional levels to modulate learning processes. And, on a molecular pathway scale, we seek to identify the network patterns that could suggest which broken functional modules are responsible for memory aberrations in neurodegenerative diseases. Since general paradigms of regeneration and degeneration can be significantly inspired by developmental biology models, we study regulatory patterns of tissue differentiation in normal and cancer conditions.
Our mission in translation and network medicine is to adopt advanced machine learning and network science approaches to integrate molecular networks and omic profiles for the definition of personalised therapeutic plans and individualised drug treatments. Furthermore, as the cardiovascular system is a paradigmatic example of an adaptive complex system, we apply our pattern recognition algorithms to explore normal/pathological conditions in cardiovascular patients.
Finally, since the CCNI aims to study the complexity of life systems and their mechanisms of adaptation across scales, we collaborate with experts in ecological, social and economic science in order to apply our algorithms to their data and to reveal whether generalized rules of self-organization characterize life matter from molecules and cells to microbes and animals (including humans).
Degrees
Italian Inter-polytechnic School of Doctorate (Turin, Milan and Bari), PhD, 2010.
Polytechnic School of Milan, Milan, Italy, MS, 2005.
Previous Appointments
Biotechnologisches Zentrum (BIOTEC), Center for Molecular and Cellular Bioengineering (CMCB), Technische Universität Dresden (TUD), Germany. Principal Investigator (Tenured),2019 -2020
Biotechnologisches Zentrum (BIOTEC), Center for Molecular and Cellular Bioengineering (CMCB), Technische Universität Dresden (TUD), Germany. Principal Investigator (Tenure-track), 2014-2018
King Abdullah University of Science and Technology (KAUST), Jeddah, Saudi Arabia. Researcher Scientist, 2013
King Abdullah University of Science and Technology (KAUST), Jeddah, Saudi Arabia. Postdoctoral Researcher, 2010-2012
University of California, San Diego, USA. Visiting Scholar, 2009
San Raffaele Scientific Institute, Milan, Italy. Research Fellow, 2006-2010
Biomedical Engineering Institute of the Italian National Research Council (ISIB-CNR, Milan department), Milan, Italy. Research Fellow, 2005-2006
Selected Publications
Artificial Intelligence & Network Science & Physics
Neuromorphic Dendritic Computation with Silent Synapses for Visual Motion Perception
Eunye Baek, Sen Song, Zong Rong, Luping Shi, Carlo Vittorio Cannistraci
Nature Electronics, 2024
Epitopological learning and Cannistraci-Hebb network shape intelligence brain-inspired theory for ultra-sparse advantage in deep learning
Yingtao Zhang, Jialin Zhao, Wenjing Wu, Alessandro Muscoloni, Carlo Vittorio Cannistraci
The Twelfth International Conference on Learning Representations (ICLR) 2024
Plug-and-Play: An Efficient Post-training Pruning Method for Large Language Models
Yingtao Zhang, Haoli Bai, Haokun Lin, Jialin Zhao, Lu Hou, Carlo Vittorio Cannistraci
The Twelfth International Conference on Learning Representations (ICLR) 2024
Stealing fire or stacking knowledge’ by machine intelligence to model link prediction in complex networks
Alessandro Muscoloni and Carlo Vittorio Cannistraci
iScience, 2023
Geometrical congruence, greedy navigability and myopic transfer in complex networks and brain connectomes
Carlo Vittorio Cannistraci and Alessandro Muscoloni
Nature Communications, 2022
Nonlinear machine learning pattern recognition and bacteria-metabolite multilayer network analysis of perturbed gastric microbiome
Claudio Duran, …, Giovanni Gasbarrini, Antonio Gasbarrini & Carlo Vittorio Cannistraci
Nature Communications, 2021
Modular gateway-ness connectivity and structural core organization in maritime network science
Mengqiao Xu, Qian Pan, Alessandro Muscoloni, Haoxiang Xia & Carlo Vittorio Cannistraci
Nature Communications, 2020
Intrinsic plasticity of silicon nanowire neurotransistors for dynamic memory and learning functions
Eunhye Baek, Nikhil Ranjan Das, Carlo Vittorio Cannistraci, … & Gianaurelio Cuniberti
Nature Electronics, 2020
Navigability evaluation of complex networks by greedy routing efficiency
Alessandro Muscoloni & Carlo Vittorio Cannistraci
Proceedings of the National Academy of Sciences, 2019
A nonuniform popularity-similarity optimization (nPSO) model to efficiently generate realistic complex networks with communities
Alessandro Muscoloni & Carlo Vittorio Cannistraci
New Journal of Physics, 2018
Machine learning meets complex networks via coalescent embedding in the hyperbolic space
Alessandro Muscoloni, … , Ginestra Bianconi & Carlo Vittorio Cannistraci
Nature Communications, 2017
Common neighbours and the local-community-paradigm for topological link prediction in bipartite networks
Simone Daminelli, Josephine Maria Thomas, Claudio Durán & Carlo Vittorio Cannistraci
New Journal of Physics, 2015
From link-prediction in brain connectomes and protein interactomes to the local-community-paradigm in complex networks
Carlo Vittorio Cannistraci, Gregorio Alanis-Lobato, Timothy Ravasi
Scientific reports, 2013
Computational Biomedicine & Neuroscience
Spatial Reconstruction of Oligo and Single Cells by De Novo Coalescent Embedding of Transcriptomic Networks
Y Zhao, S Zhang, J Xu, Y Yu, G Peng, CV Cannistraci, JDJ Han
Advanced Science, 2023
Prevalence, Characteristics, and Outcomes of COVID-19–Associated Acute Myocarditis
E Ammirati, L Lupi, M Palazzini, NS Hendren, JL Grodin, CV Cannistraci, …, Marco Metra
Circulation, 2022
Proprotein convertase subtilisin/kexin 9 (PCSK9) promotes macrophage activation via LDL receptor-independent mechanisms
Shunsuke Katsuki, ... , Carlo V. Cannistraci, ... , Masanori Aikawa
Circulation Research, 2022
Three-dimensional facial-image analysis to predict heterogeneity of the human ageing rate and the impact of lifestyle
Xian Xia, … , Carlo Vittorio Cannistraci, Yong Zhou & Jing-Dong J Han.
Nature Metabolism, 2020
Machine learning of human plasma lipidomes for obesity estimation in a large population cohort
Mathias J Gerl, ... , Carlo Vittorio Cannistraci and Kai Simons
Plos Biology, 2019
Pioneering topological methods for network-based drug–target prediction by exploiting a brain-network self-organization theory
Claudio Durán, Simone Daminelli, ..., & Carlo Vittorio Cannistraci
Briefings in Bioinformatics, 2018
A promoter-level mammalian expression atlas
Fantom Consortium (including Carlo Vittorio Cannistraci)
Nature, 2014
Differential roles of epigenetic changes and Foxp3 expression in regulatory T cell-specific transcriptional regulation
Fantom Consortium (including Carlo Vittorio Cannistraci)
Proceedings of the National Academy of Sciences, 2014
Minimum curvilinearity to enhance topological prediction of protein interactions by network embedding
Carlo Vittorio Cannistraci, Gregorio Alanis-Lobato, Timothy Ravasi
Bioinformatics, 2013
Identification and Predictive Value of Interleukin-6+ Interleukin-10+ and Interleukin-6− Interleukin-10+ Cytokine Patterns in ST-Elevation Acute Myocardial Infarction
Enrico Ammirati^, Carlo Vittorio Cannistraci^, … & Attilio Maseri
Circulation Research, 2012 (^first co-authorship)
Nonlinear dimension reduction and clustering by Minimum Curvilinearity unfold neuropathic pain and tissue embryological classes
CV Cannistraci, T Ravasi, FM Montevecchi, T Ideker, M Alessio
Bioinformatics, 2010
An atlas of combinatorial transcriptional regulation in mouse and man
Timoty Ravasi^, Harukazu Suzuki^, Carlo Vittorio Cannistraci^, et al.
Cell, 2010 (^first co-authorship)
Honors & Awards
Member of the Network Science Society https://netscisociety.net/home.
Member of the International Society for Computational Biology https://www.iscb.org/
2024 Chair of the section on frontiers of algorithms and technologies of the Cold Spring Harbor Asia conference on Computational Biology of the Genome.
2024, Associated Editor of Applied Network Science https://appliednetsci.springeropen.com/
2023 Chair of the Network Geometry section in the International School and Conference on Network Science.
2023 Chair of the Temporal Network Section in the 12th International Conference on Complex Networks and their applications
2023 Section Editor for Concepts in Complexity in Plos Complex Systems,l till September 2024.
2023 Committee Representative Network Biology (NetBio, http://cosi.iscb.org/wiki/NetBio:Home) Community of special interest (COSI) for the International Society Computational Biology (ISCB) from February 2023 to January 2024.
Selected Editor (6 selected in total) for the 10th Anniversary Editorial Board Interviews of Scientific Reports https://www.nature.com/srep/highlights/anniversary-interviews.
2021, National high-level senior talent award from the Minister of Science of China.
2020, Chair Professorship award of Tsinghua University.
2019, Shanghai 1000 talents plan award
2019, Scientific Reports selected interview to represent the journal in the social media.
2018, Chair for Keynote speaker section of the 7th International Conference on Complex Networks and Their Applications 2018, Cambridge, UK
2017, Chair for the network model section of the 6th International Conference on Complex Networks and Their Applications 2017, Lyon, France
2016, Chair for the biological network section of the 5th International Conference on Complex Networks and Their Applications 2016, Milano, Italy
2016, Technische Universität Dresden (TUD) Young Investigator Award in Physics.
Contact Informations
Email: kalokagathos.agon@gmail.com or kailong@mail.tsinghua.edu.cn
Office: Chengfu Road 160, Haidian District, Beijing
Phone: +86-10-62783675